In May, ProPublica released a groundbreaking article titled “Machine Bias,” authored by Julia Angwin and Jeff Larson. This eye-opening piece delved into the world of algorithmic bias, shedding light on the startling disparities found in recidivism risk models. Recidivism risk models are tools used in the criminal justice system to assess the likelihood of a defendant returning to prison. The article’s revelations regarding racial bias in these models have far-reaching implications for the fairness and transparency of the judicial process.
Understanding Recidivism Risk Models
Recidivism risk models stand at the intersection of data analytics and criminal justice, serving as essential tools in predicting the probability of a convicted individual returning to a life of crime. These intricate algorithms harness a comprehensive array of inputs, encompassing not only an individual’s criminal history but also delving into demographic information, socioeconomic status, and even behavioral patterns. The resultant risk scores derived from this complex analysis wield substantial influence within the criminal justice system, impacting crucial decisions such as sentencing, considerations for bail, and determinations related to parole eligibility. In essence, these models represent a multidimensional approach to understanding and addressing the dynamics of repeat offending, seeking to strike a balance between rehabilitation and public safety.
Key Findings of the ProPublica Report
Angwin and Larson conducted an in-depth examination of the recidivism risk model known as COMPAS. To assess its accuracy, they analyzed COMPAS scores for 10,000 criminal defendants in Broward County, Florida, comparing predictions with actual outcomes. The results exposed significant disparities:
- Racial Disparities in Risk Assessment: Black defendants were often assigned higher recidivism risk scores than their actual likelihood of recidivism. Shockingly, black defendants who did not re-offend over a two-year period were nearly twice as likely to be misclassified as higher risk compared to white counterparts (45 percent vs. 23 percent);
- Racial Bias Against White Defendants: Conversely, white defendants were often predicted to be less risky than they were in reality. White defendants who re-offended within two years were mistakenly labeled as low risk almost twice as often as black re-offenders (48 percent vs. 28 percent);
- Persistent Racial Bias: Even after controlling for factors such as prior crimes, future recidivism, age, and gender, black defendants were 45 percent more likely to receive higher risk scores than white defendants;
- Violent Recidivism Bias: The bias extended to predictions of violent recidivism, where black defendants were twice as likely to be misclassified as higher risk than white defendants. White violent recidivists were also 63 percent more likely to be misclassified as low risk compared to black violent recidivists.
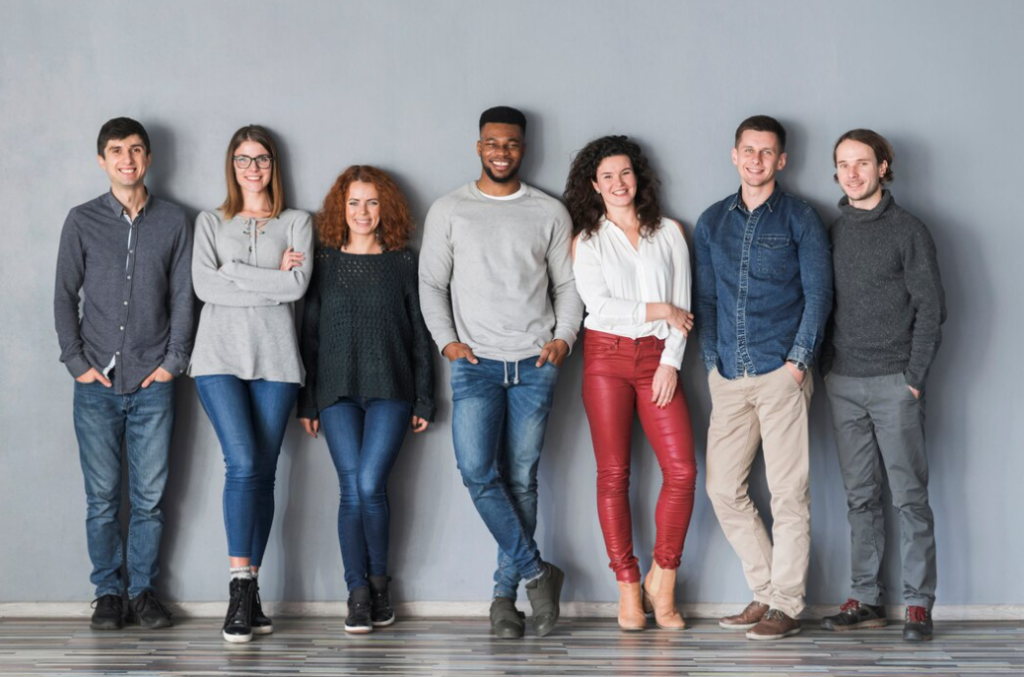
ProPublica’s Methodology
ProPublica’s commitment to data journalism standards was evident throughout their investigation. They published their methodology, including a comprehensive review of prior studies on racial disparities in recidivism risk scoring models. Importantly, they made their data and analysis available on GitHub for public scrutiny.
Their research relied heavily on open records laws in Florida, granting access to crucial data, such as original scores, subsequent arrest records, and racial classifications. This transparency allowed them to build a robust analysis, tracking both recidivism and violent recidivism, as well as the original scores and error rates.
ProPublica’s investigation marks a significant milestone for advocates concerned about algorithmic bias in criminal justice. While previous arguments in this area lacked hard evidence, this report provides concrete proof of racial bias in recidivism risk models. This breakthrough is not only valuable for highlighting the issue but also sets a benchmark for future analyses.
The transparency demonstrated by ProPublica in their investigation is vital. It ensures that nobody can claim ignorance about the computation of these statistics, making it a fundamental benchmark for assessing all recidivism risk models. However, it’s important to note that the standard for optimizing these algorithms should not be limited to false negatives and false positives.
The Consequences of Racial Bias in Recidivism Risk Models
The presence of racial bias in recidivism risk models carries significant and far-reaching implications that reverberate throughout the criminal justice system and society at large, extending well beyond the mere technical aspects of algorithmic design:
- Injustice and Disproportionate Sentencing: Racial bias within these models perpetuates systemic injustices by leading to disproportionately harsh sentences for minority defendants. This can, in turn, result in the tragic occurrence of wrongful convictions, where individuals may be penalized more severely based on their racial background rather than the merits of their case. This inherent unfairness within the system underscores the urgent need for reform;
- Undermining Trust and Public Confidence: Beyond its direct impact on individuals, the perception of racial bias in recidivism risk models erodes public trust in the entire criminal justice system. When people believe that the system is inherently biased, it fosters a sense of unfairness and inequality, ultimately undermining the system’s credibility and effectiveness. Rebuilding this trust becomes an essential endeavor for maintaining a just and functioning society;
- Reinforcing Negative Stereotypes: Biased algorithms not only harm individuals but also contribute to the reinforcement of negative stereotypes. By perpetuating these stereotypes, they further marginalize minority communities and hinder progress towards a more inclusive and equitable society. It is imperative to recognize and confront this harmful cycle to break free from ingrained biases and prejudices.
Algorithmic Bias: A Broader Perspective
Algorithmic bias, as exemplified by the ProPublica report, transcends its immediate context to become a pervasive issue that infiltrates numerous aspects of our modern existence. It casts a wide and unsettling net, extending from the realms of criminal justice into domains as diverse as finance and healthcare. To fully grapple with this complex problem, it’s imperative to broaden our perspective and recognize its multifaceted nature.
- Data Bias Beyond Borders: A fundamental contributor to algorithmic bias is the data on which these systems are trained. Often, this data reflects the historical biases and prejudices of human decision-making. When such biased datasets are utilized to train machine learning models, the algorithms inherit and perpetuate these ingrained biases, effectively cementing them into automated decision-making processes;
- Complexity and Opaqueness: Algorithmic decision-making processes can be breathtakingly intricate and, at times, maddeningly opaque. The inner workings of these algorithms can resemble enigmatic “black boxes,” where inputs and outputs are visible, but the intricate mechanics in-between remain shrouded. This opacity adds another layer of complexity to the challenge of identifying and rectifying bias, making it an arduous task for stakeholders seeking algorithmic fairness;
- Unequal Impact: The consequences of algorithmic bias are not evenly distributed. Marginalized groups bear a disproportionate burden of the harm caused by biased algorithms. These systems tend to amplify existing inequalities and injustices, further marginalizing and disadvantaging the very groups that are already vulnerable;
- Accountability Dilemma: Establishing clear lines of accountability in the realm of algorithmic bias is a multifaceted puzzle. Responsibility often straddles the developers who create these algorithms and the users who deploy them. It becomes an intricate dance of defining who should be held accountable for the biases that emerge in algorithmic decision-making, raising challenging questions about regulation and oversight.
Solutions to Address Algorithmic Bias
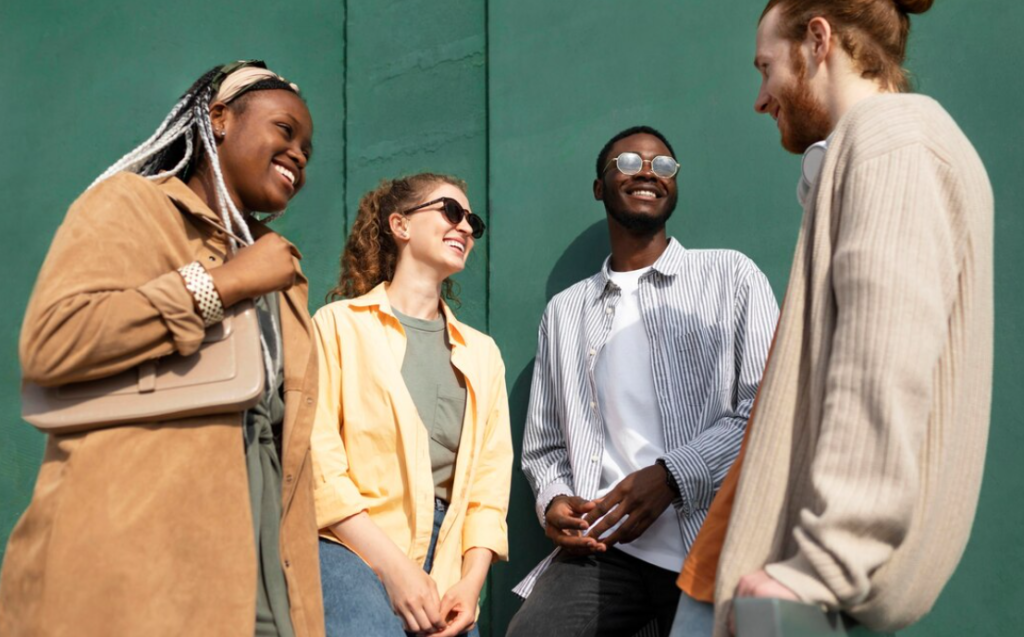
Addressing algorithmic bias is a multifaceted challenge that requires a concerted effort from various stakeholders. Here are some potential solutions and strategies:
- Diverse Data: Ensure that data used to train algorithms are diverse, representative, and regularly audited for bias. Efforts should be made to collect and include data from underrepresented groups to counteract the historical biases ingrained in existing datasets. A more inclusive data foundation can help algorithms make fairer predictions and decisions;
- Transparency: Promote transparency in algorithmic decision-making processes. Organizations should not treat their algorithms as mysterious “black boxes.” Instead, they should disclose how their algorithms work, what data they use, and how decisions are made. Transparency is essential for understanding and mitigating bias;
- Fairness Assessments: Conduct fairness assessments during the development and deployment of algorithms to identify and rectify bias. Use metrics that measure fairness across different demographic groups, ensuring that algorithms don’t disproportionately impact any particular group. Regular audits should be part of the algorithm’s lifecycle;
- Regulation and Legislation: Governments and regulatory bodies should consider legislation that addresses algorithmic bias and enforces transparency and accountability in algorithm development and use. Legal frameworks can provide clear guidelines and consequences for organizations that fail to address bias adequately;
- Ethical AI Practices: Encourage the adoption of ethical AI practices within organizations. This includes the creation of diverse and interdisciplinary teams to develop and assess algorithms. By including a variety of perspectives, organizations can better identify and rectify bias in their systems;
- Algorithmic Auditing: Establish independent auditing mechanisms to evaluate the fairness and impact of algorithms used in critical domains like criminal justice. These auditors can provide impartial assessments, helping to ensure that algorithms meet fairness standards and don’t perpetuate bias.
Conclusion
The ProPublica report, “Recidivism Risk Models: Racially Biased?” has raised important questions about the fairness and equity of recidivism risk models within the criminal justice system. It highlights the pressing need for transparency, accountability, and ongoing reform to ensure that these models do not perpetuate racial bias. As discussions continue, it is imperative to prioritize the creation of a just and equitable criminal justice system that is blind to race and ethnicity.