In the rapidly evolving landscape of financial technology, algorithms are becoming the cornerstone of decision-making processes. They determine who gets a loan, who gets a credit card, and even who gets access to certain services. However, as these algorithms gain prominence, a critical issue has emerged: algorithmic bias. This problem came into sharp focus when the Apple Card, launched in August, became embroiled in a controversy that highlighted the challenges and complexities of addressing bias in AI-driven financial decisions.
The Controversy Unfolds
The Apple Card controversy didn’t quietly emerge; it erupted with a resounding crescendo that reverberated through the financial technology industry. It all began when users of the Apple Card started noticing a deeply troubling pattern: the credit limits extended to women were significantly lower than those granted to their male counterparts. What made this issue all the more disconcerting was its pervasiveness; it was not a sporadic occurrence but a systematic bias that affected a substantial number of users.
As word of this disparity spread across social media platforms, particularly on the vocal and influential Twitter community, the collective outcry grew into a deafening chorus.
- The hashtag #AppleCardBias became a rallying cry for those who were disheartened by what they perceived as gender discrimination embedded within the very fabric of a financial product;
- In the court of public opinion, the Apple Card was swiftly branded as “fucking sexist” and “beyond f’ed up.”;
- Even Steve Wozniak, Apple’s co-founder, known for his amiable disposition, expressed his concerns about the card, albeit in a more polite tone, wondering whether it might harbor misogynistic tendencies.
However, the reverberations of this controversy did not stop at social media outrage. The outcry reached the ears of regulatory authorities on Wall Street, who recognized the need for a comprehensive investigation. A Wall Street regulator, acknowledging the gravity of the situation, announced its intent to probe the inner workings of the Apple Card. The objective was to determine whether the card’s algorithmic decision-making processes ran afoul of any established financial regulations. With regulatory scrutiny looming, both Apple and Goldman Sachs, the issuing bank for the Apple Card, found themselves thrust into a harsh spotlight, subjected to intense scrutiny and criticism from all quarters.
The Defense and Its Limitations
In the wake of the Apple Card gender bias allegations, Goldman Sachs found itself at the forefront, tasked with providing a staunch defense against the accusations. With conviction, they asserted that the algorithm responsible for determining credit limits was devoid of any gender bias. Moreover, they emphasized that this algorithm had undergone a rigorous examination for potential bias by an independent third party. In an attempt to reassure the public, they boldly stated that gender played no role in shaping the outcomes of the algorithm. On the surface, these declarations appeared robust, constituting a formidable defense against the troubling claims of gender bias.
Yet, as the controversy unfolded, this explanation was met with widespread skepticism, and this skepticism was grounded in sound reasoning. While Goldman Sachs adamantly asserted that the algorithm operated in a gender-blind manner, experts and the general public raised a critical concern—a concern rooted in a fundamental understanding of algorithmic behavior.
- The crux of the issue lies in the paradox of gender-blind algorithms;
- These algorithms are meticulously engineered to exclude gender as an explicit input variable, with the intent of preventing any form of bias;
- However, as the controversy revealed, the mere act of removing gender as an input does not guarantee impartiality;
- This realization struck at the heart of the matter and cast doubt on the prevailing notion that algorithms can be entirely free from bias.
The skepticism arose from a fundamental truth: algorithms can inadvertently perpetuate bias even when they are programmed to be “blind” to variables like gender. This phenomenon stems from the subtle interplay of correlated inputs, proxy variables, and the intricacies of algorithmic decision-making. In other words, an algorithm can indirectly introduce gender bias by relying on seemingly unrelated factors that correlate with gender. For instance, creditworthiness might be predicted using entirely neutral criteria, such as the type of computer one uses. Yet, if computer choice correlates with gender, it can introduce gender bias into the algorithm’s outcomes.
The skepticism that shrouded Goldman Sachs’ defense served as a stark reminder of the complexity of algorithmic bias and the limitations of relying solely on the absence of explicit input variables as a safeguard against discrimination. It underscored the need for a deeper understanding of how algorithms operate and the imperative of proactive measures to detect and mitigate bias, even in algorithms designed to be “blind” to certain attributes.
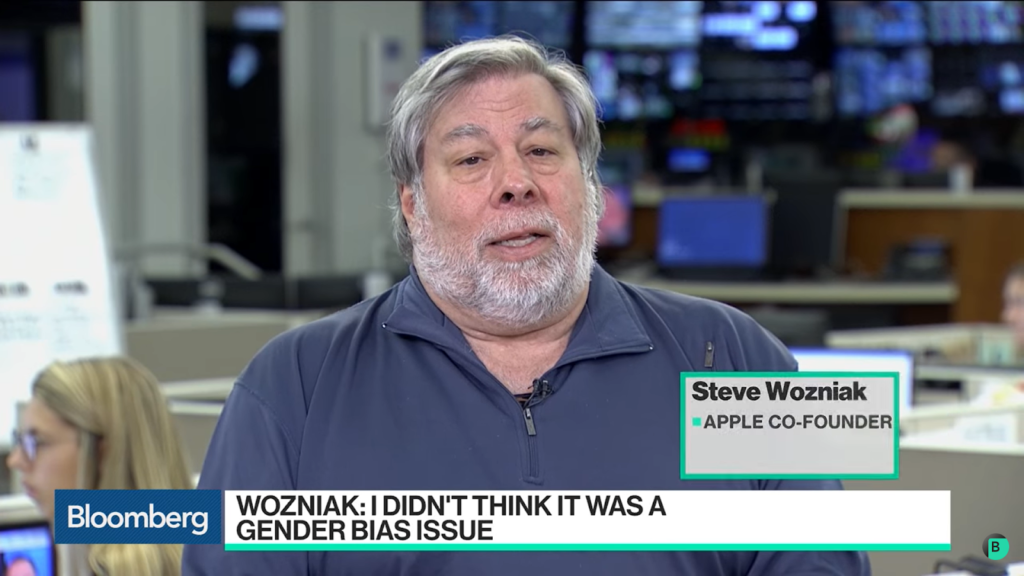
The Misleading Notion of Gender-Blind Algorithms
The concept of “gender-blind” algorithms, while born out of a genuine desire to mitigate bias, reveals itself to be a double-edged sword upon closer examination. At its core, these algorithms are meticulously crafted to exclude gender as an explicit input in a commendable effort to prevent bias from seeping into decision-making processes. However, what becomes strikingly evident is that this noble intention doesn’t necessarily translate into a guarantee of fairness. The complexity of algorithmic behavior often unveils a less straightforward reality, where the absence of gender as an input does not automatically shield the system from discriminatory outcomes. This nuanced paradox is rooted in a concept known as proxy variables.
Proxy variables are the unsuspecting conduits through which gender bias can surreptitiously infiltrate ostensibly gender-neutral algorithms. These variables are seemingly unrelated to gender on the surface, yet they bear the clandestine ability to indirectly perpetuate gender-based discrimination within the algorithm’s outcomes.
To illustrate this point, consider a seemingly innocuous factor like the type of computer one uses. In isolation, it may appear entirely unrelated to an individual’s gender. However, within the vast sea of data, correlations can emerge. If, for instance, certain computer choices are more prevalent among one gender than another, the algorithm may unwittingly incorporate this seemingly neutral variable into its decision-making process. Consequently, it can indirectly introduce gender bias into the outcomes, even though gender was intentionally excluded as a direct input.
The complexities don’t end here. Variables as seemingly mundane as home address can inadvertently serve as proxies for an individual’s race, and shopping preferences can inadvertently overlap with information related to gender. These subtle and intricate correlations between ostensibly neutral variables and protected attributes represent a minefield of potential bias in algorithmic decision-making. They underscore the multifaceted nature of algorithmic fairness and the need for a deeper understanding of how seemingly unrelated factors can intersect with sensitive attributes, ultimately impacting the equitable treatment of individuals in automated systems.
The Importance of Recognizing Proxies and Addressing Bias
The pervasive influence of proxies in perpetuating algorithmic bias is a challenge that extends far beyond the realm of finance. It casts a long shadow over various domains, including education, criminal justice, and healthcare. The insidious nature of proxies becomes increasingly apparent as they play a significant role in shaping automated systems, often resulting in outcomes that are both unfair and biased. Recognizing the profound significance of proxies in the context of algorithmic bias is a crucial step toward effectively tackling this pervasive issue.
The Apple Card controversy, while a prominent and well-publicized example, serves as a poignant reminder of the broader problem at hand. It underscores the urgency of meticulously auditing algorithms to detect and rectify bias, irrespective of the specific domain in which they operate. The notion that a lack of explicit data equates to fairness is a fallacy that the controversy has effectively debunked. Blindly assuming that algorithms are impartial because certain attributes are excluded from explicit consideration is a perilous oversight.
Instead, companies and organizations must embrace a proactive approach to address bias head-on. This entails actively measuring protected attributes like gender and race, even when this data is not explicitly provided by users. Rather than relying solely on readily available data, such as shopping preferences or device choices, recognizing the importance of directly assessing protected attributes is paramount. It ensures that potential bias, whether intentional or inadvertent, is identified and addressed comprehensively.
Furthermore, the quest for algorithmic fairness necessitates the involvement of legal and technical experts, particularly in the post-deployment phase. While the initial design of algorithms strives to be equitable, the real test comes when these systems are put into practice. Legal and technical experts play a crucial role in monitoring algorithms for unintended bias, ensuring that fairness and transparency remain at the forefront of automated decision-making processes. Their expertise becomes indispensable in identifying and rectifying issues that may arise as algorithms interact with real-world data and users.
Conclusion
The Apple Card controversy underscores the multifaceted nature of algorithmic bias and the challenges involved in mitigating it. As technology continues to shape our financial lives, we must remain vigilant in addressing biases that can inadvertently permeate these systems. By recognizing the limitations of “blind” algorithms and actively seeking ways to identify and rectify bias, we can work toward creating a more equitable and inclusive financial landscape. The future of finance depends on our ability to navigate the complex interplay between technology, fairness, and society.
While exploring the multifaceted challenges of algorithmic bias in technology, you may also like to consider O’Neil’s Take on Big Data’s Threat to Democracy, where similar concerns about the impact of technology on our society and democracy are discussed.